Google DeepMind has a new AI model that can direct robotic tasks it was never trained to perform.
Named RT-2, the model learns from web and robotics data. It then turns this information into simple instructions for machines.
In tests, the model was asked to take actions never seen in the robotic data, such as placing oranges in a matching bowl. To follow these commands, the system had to translate knowledge from web-based data. According to DeepMind, the model had a 62% success for these operations — double that of its predecessor, RT-1.
“Just like language models are trained on text from the web to learn general ideas and concepts, RT-2 transfers knowledge from web data to inform robot behaviour,” said Vincent Vanhoucke, head of robotics at DeepMind. “In other words, RT-2 can speak robot.”
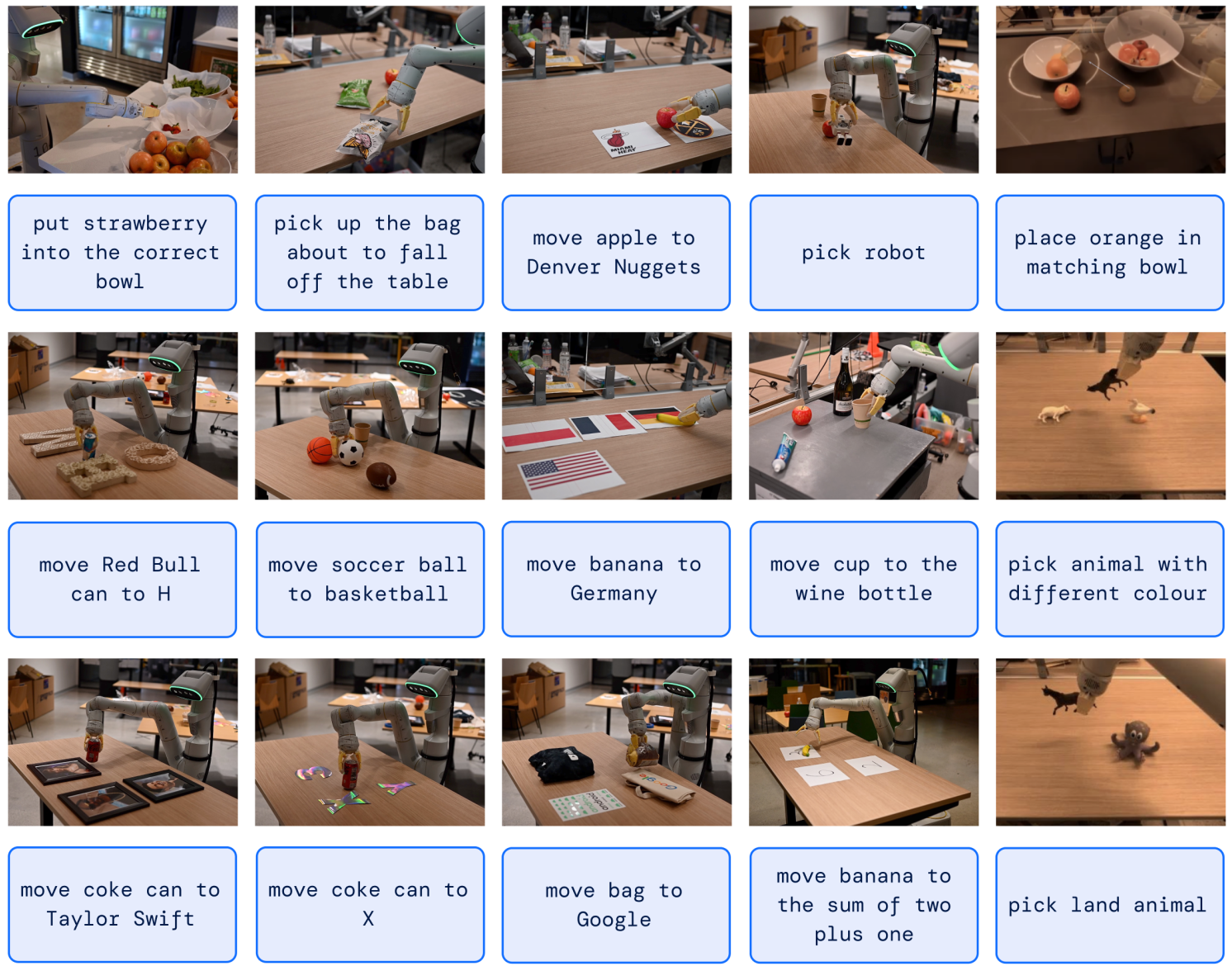
The tests showed RT-2 has impressive generalisation capabilities. It also has an improved semantic and visual understanding of robotic data that wasn’t previously encountered.
Notably, the model can use rudimentary reasoning to follow new user commands. Impressively, it can even perform multi-stage semantic reasoning. For instance, when instructed to pick an object that could be used as a hammer, RT-2 correctly identified a rock as the best option.
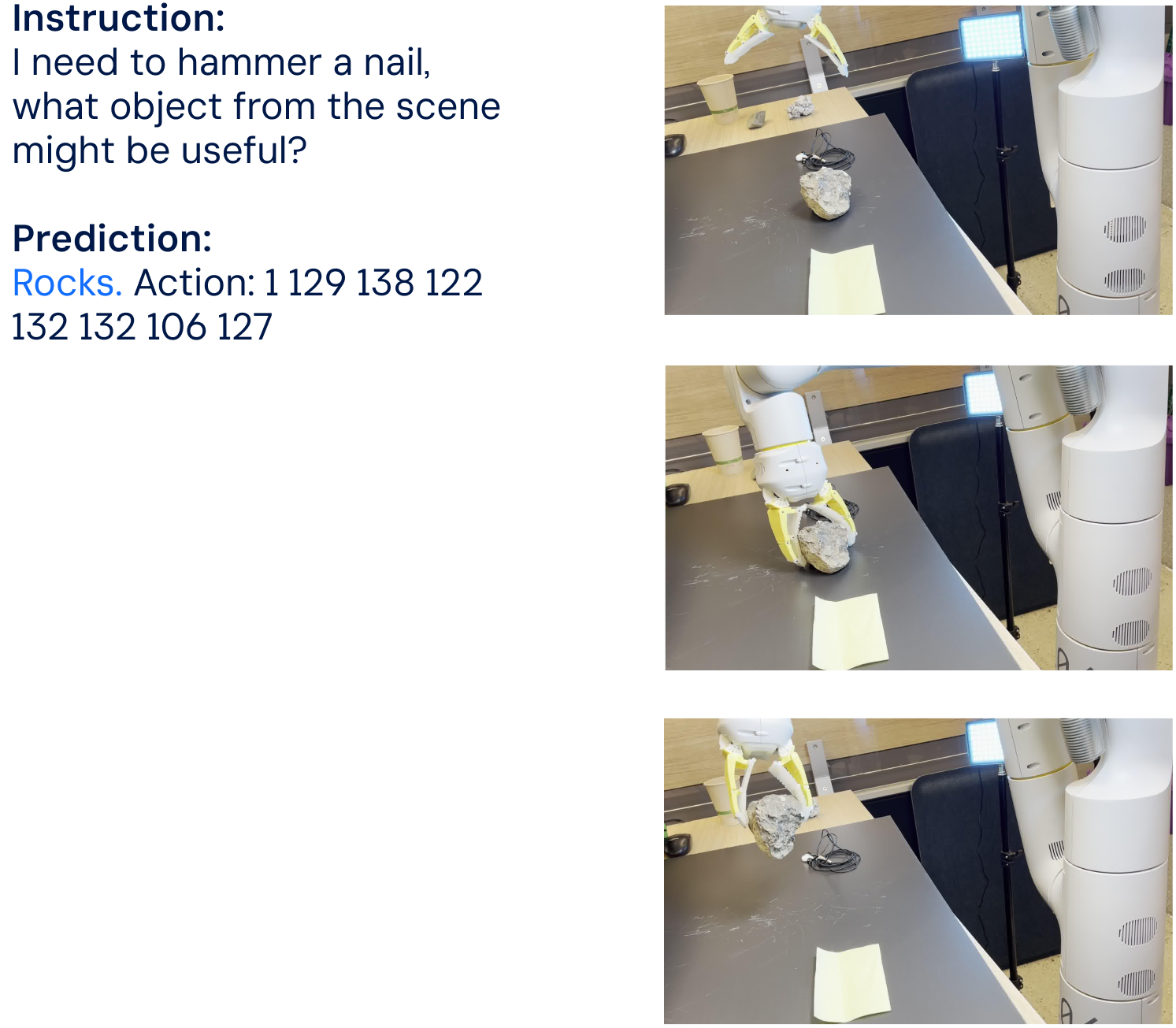
In another evaluation, the model was commanded to push a bottle of ketchup towards a blue cube.
There were several items in the scene, but the only one in the training dataset was the cube. Nonetheless, RT-2 successfully pushed the ketchup towards the specified destination.
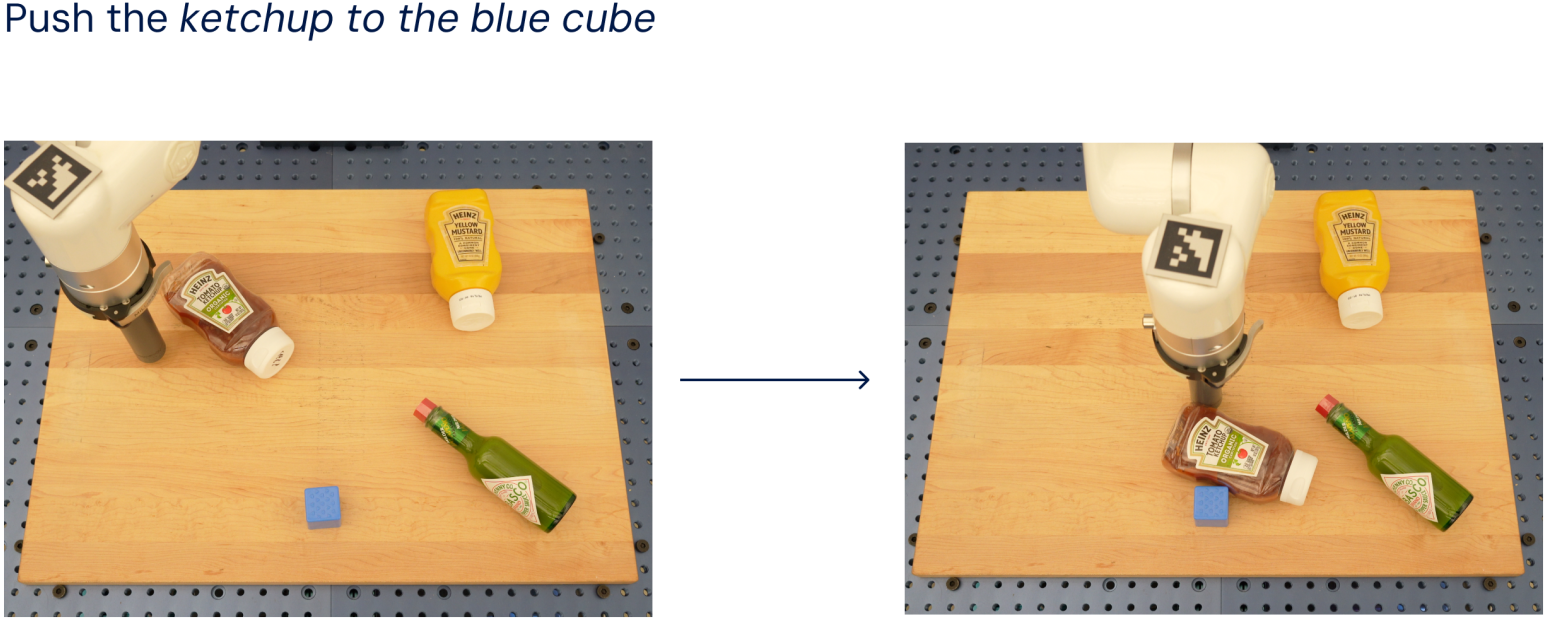
DeepMind has heralded RT-2 as a breakthrough in artificial intelligence. The Londonlab says the model brings us closer to a future of helpful robots.
“Not only does RT-2 show how advances in AI are cascading rapidly into robotics, it shows enormous promise for more general-purpose robots,” said Vanhoucke. “While there is still a tremendous amount of work to be done to enable helpful robots in human-centered environments, RT-2 shows us an exciting future for robotics just within grasp.”
You can read the RT-2 study paper here.
Published