An Italian company has unveiled a novel method of measuring AI progress: analyzing improvements in machine translation.
Translated, a provider of translation services, used the approach to predict when we will achieve singularity, a vague concept often defined as the point where machines become smarter than humans.
The Rome-based business sets this milestone at the moment when AI provides “a perfect translation.” According to the new research, this arrives when machine translation (MT) is better than top human translations.
Translated’s analysis suggests this will happen before the end of the 2020s.
“[It will be] within this decade, at least for the top 10 languages in a context of average complexity,” Marco Trombetti, the company’s CEO, tells TNW. “The reality is that in some specific domains and in a few languages this has already happened. For some rare languages and domains it may never come.”
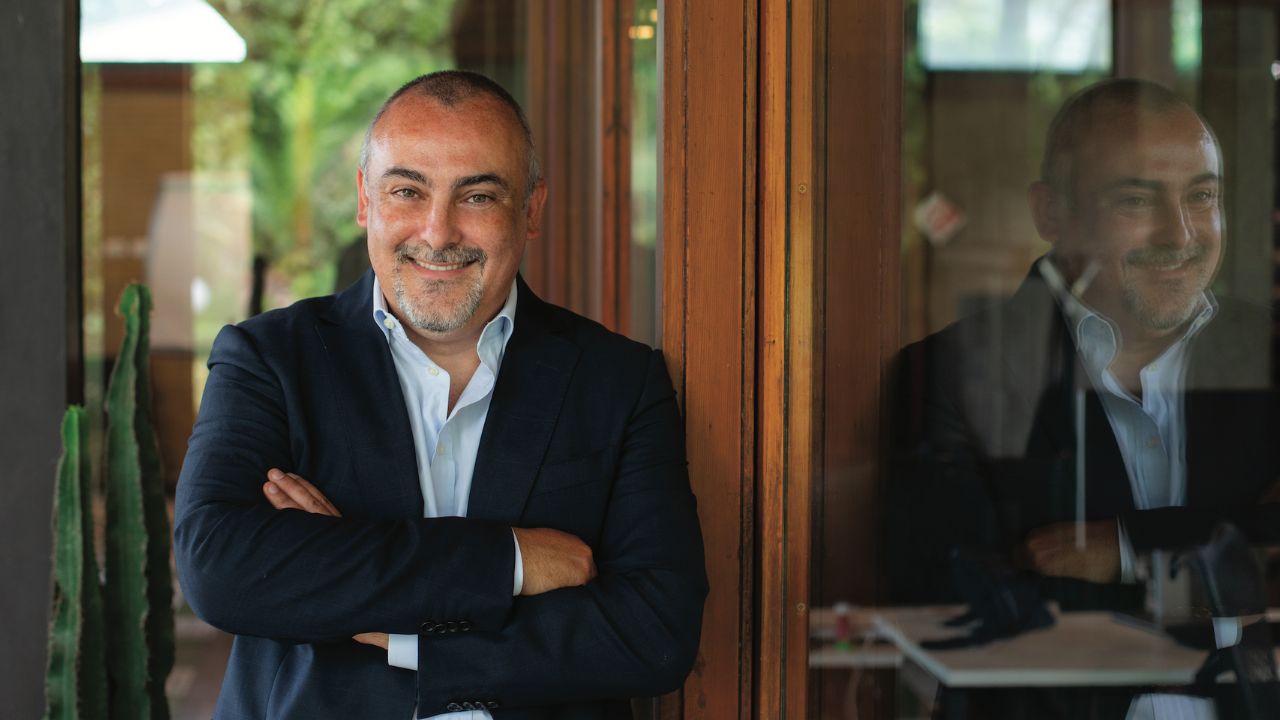
Translated’s estimates are based on data taken from Matecat, a computer-assisted translation (CAT) tool.
The platform began life in 2011 as an EU-funded research project. Three years later, the system was released as open-source software, which professionals use to improve their translations.
Translated offers Matecat as a freemium product. In return, users provide the company with data that’s used to improve its models.
To chart the path to singularity, Translated tracked the time users spent checking and correcting 2 billion MT suggestions. Around 136,000 professionals worldwide had made these edits across Matecat’s 12 years of operation. The translations spanned diverse domains, from literature to technical subjects. They also included fields in which MT is still struggling, such as speech transcription.
“Singularity is really close.
The data suggests that AI is rapidly improving. In 2015, the average time that world-leading translators took to check and correct MT suggestions was around 3.5 seconds per word. Today, that number’s down to 2 seconds per word.
At the current rate, the time will hit 1 second in around five years. At that point, MT would provide the epochal “perfect translation.” In practical terms, it will then be more convenient to edit a machine’s translations than a top professional’s.
According to Trombetti, any task involving communication, understanding, listening, and sharing knowledge will become multilingual with minimal investment.
“The exact date of when we will reach the singularity point may vary, but the trend is clear: it is really close,” he says.
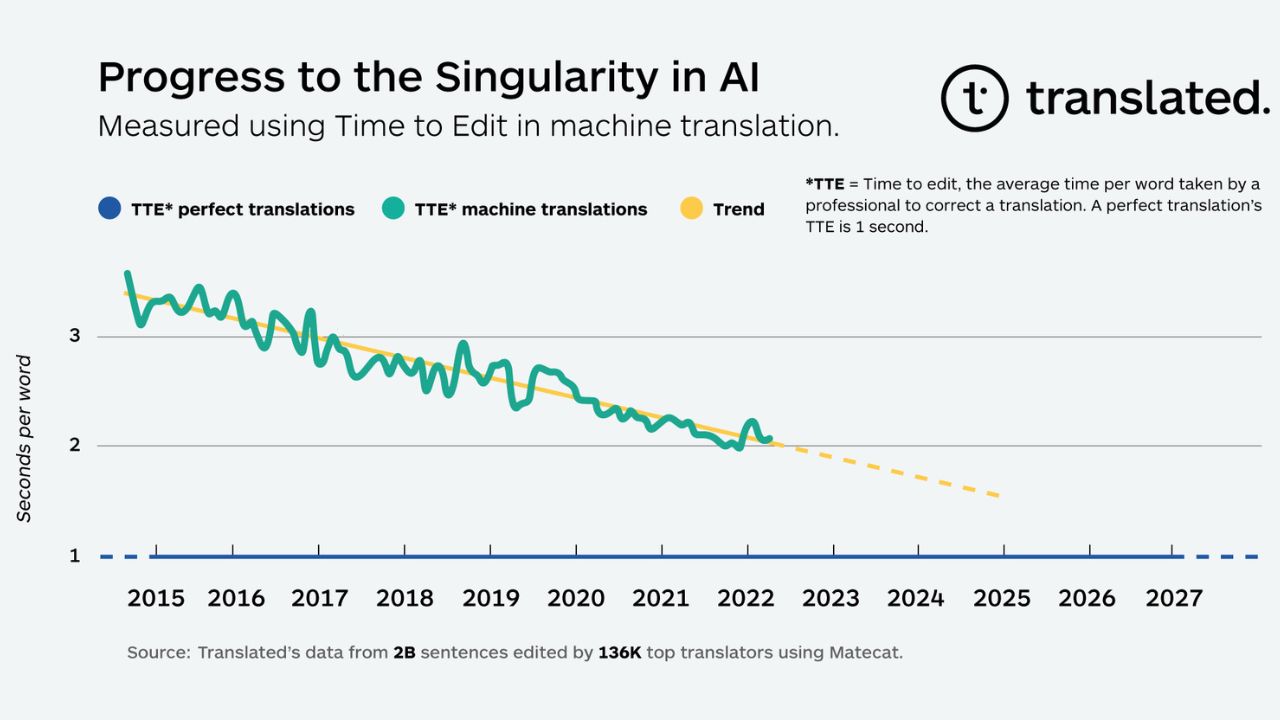
Advances in MT require increasing computing power, linguistic data, and algorithmic efficiency. Consequently, the researchers had presumed progress would slow as singularity approached. To their surprise, the rate of development was highly linear.
If this momentum continues as predicted, Translated anticipates demand for MT to be at least 100 times higher. Workers may worry that their jobs will be automated, but they could also benefit. Translated forecasts at least a tenfold increase in requests for professional translations.
“All our customers who are deploying machine translation on a large scale are also spending more on human translation,” says Trombetti.
“Machine translation is an enabler in that it creates more interactions between markets and users that were not in contact before. This generates business, and business generates higher-quality content that requires professionals.”
Trombetti also expects new roles to emerge for elite translators.
“To get the best quality out of machine translation you need it to be trained by the best linguists. A significant volume of translations is required to train language models and fix errors in them, so I guess it’s likely that we’ll witness huge competition for the best translators in the upcoming years.”
“MT is a good predictor of what’s next in AI.
According to Translated, the new research is the first to ever quantify the speed at which we’re approaching singularity. The claim won’t convince every cynic, but MT is a compelling barometer for AI progress.
Human languages are notoriously tricky for machines to master. The subjectivity of linguistic meaning, the constantly evolving conventions, and the nuances of cultural references, wordplay, and tone can be elusive for computers.
In translation, these complexities must be modelled and linked in two languages. As a result, algorithmic research, data collection, and model sizes are often pioneered in the field. The Transformer model, for instance, was applied to MT many years before being used in OpenAI’s GPT systems.
“MT is simply a good predictor of what is coming next in AI,” says Trombetti.
If what comes next is singularity, the Italian entrepreneur anticipates a new era for global communication.
He envisions universal translators, all content becoming globally available, and everyone able to speak their native language.
His definition of singularity may be questionable, but its appeal is undeniable.